How A.I. ACTUALLY Works: NASA Expert on Deepfakes, Education, Jobs, and the Future | with James Villarrubia and Marc Beckman
Marc Beckman: James, welcome to Some Future Day. It's so nice to see you today. How are you?
[00:02:13] James: I'm, I'm good, Mark. It's nice to see you as well.
[00:02:16] Marc Beckman: So, James, I found a quote that I think you can connect with. In fact, I believe you relate to. I'm going to read it to you, and I want you to give me your thoughts. Injustice anywhere is a threat to justice everywhere. I looked far and wide for this, and I think you could relate to it. Injustice anywhere is a threat to justice everywhere.
[00:02:39] Why the connection with this quote?
[00:02:41] James: yeah, actually, I include that quote in my, in my signature, in my emails. it's, It I think, is one of my favorite quotes, from, Martin Luther King, and I, I I think it speaks to, the sort of collective responsibility that we all have to think about not just ourselves and justice for ourselves, but justice for our neighbors, is that if our neighbors injustice goes un, you know, undealt with, that's actually bad for everyone, it's bad for society, it is bad for me, so that, you know, there's no such thing as an innocent bystander, is how I, how I think of it, is that you, You actually, you know, bystanders actually have to do get involved.
[00:03:17] It is your sort of communal responsibility. So, keeping that in mind makes me, you know, focused on my job, you know, in and out of government, a lot of social impact startups and whatnot. saying, yeah, I'm here to improve my community in a tiny bit, in a tiny way, but this is, this is my responsibility.
[00:03:33] Marc Beckman: Well, it's interesting because MLK was truly, an inspiration to all, but for me also personally, he's like really one of the most impressive humans I've ever, You know, read about or, or researched and something that you're talking about, that I think he's done a great job with, which I mean, perhaps we're lacking these days is the ability for a leader, to understand on a local level, on a community level, what all of their constituents are looking for and what their citizens I think he was like interesting because he was able to take, you know, he knew what every member of his church wanted and needed.
[00:04:12] And then he was able to like build from the ground up. you're an expert on artificial intelligence way, you know, way ahead of most people. So I'm curious with AI, do you see that? Do you see a parallel there is AI? Does AI have the ability to, move communities at a local level? That's Now let's dive into a direction that that that those local needs might want and require.
[00:04:38] Or is it more of a top down type of situation?
[00:04:43] James: before I dig in, I just want to make clear for our audience, you know, any views that I express today on this podcast are mine only, not the expressed views of NASA or any other federal organization or organization I have worked with. So, with that out of the way, I On the question of AI in sort of community involvement, I think there is, there is transformational potential for AI to tap into communities of engagement, but I think The struggle that I have is that a lot of the AI capabilities right now are still deeply centralized, and that yes, I could build an AI chatbot that, you know, that is engaging with my community, but I'm still going to be borrowing and renting sort of a core AI functionality from a big company like Google or OpenAI or Microsoft, etc.
[00:05:37] So, there is a push and pull there of how representative of that community it could be, but I do think that The tools at least have the capability of increasing engagement in your community, and maybe even sifting through the noise of a lot of community engagement to try to find those potentially injustices and say, hey, yeah, actually, you know, 10%, 50 percent of our, of our comments of our, of our community, you know, frustrations are all about this topic.
[00:06:06] so there's, there's power there to sort and sift, through, you know, of, for the community's needs, with AI.
[00:06:12] Marc Beckman: So you talk about these big entities like Google and and open AI creating, this artificial intelligence, or, or, is that training the artificial intelligence and, and, and, you know, what does that look like? What exactly, does that, does that consist of behind the scenes? Like, how is an artificial intelligence
[00:06:32] built?
[00:06:33] James: Yeah, so, what most people are probably concerned with these days is the newest generation of artificial intelligence, so generative AI, and the word People hear a lot these days is LLM or large language model. so when we're talking about those, those sorts of things, which is really what's upsetting, upsetting the world order these days.
[00:06:55] An LLM is, is basically Google or Microsoft or some very large company, OpenAI, some non profits, they go to, some other, some other third party. who has been collecting just tons of text data, tons of data from around the world, through histories, getting books. So these third parties have collected this massive corpus of information from all of mankind, humankind's, excuse me, writings.
[00:07:21] And then they, you know, sell that to a large sort of compute provider. So this is all the sort of big cloud providers, the ones really in this game. And those cloud providers set up a pretty complex, Artificial Intelligence Engine, a neural net, but it's really all it's doing is hey, I'm going to read in all that text data, I'm going to squeeze it down, into something that is a little bit more manageable of a size, but retain all the semantic and syntactic relationships, all the, all the good stuff without the specific text of the original, corpus, but maintain all the relationships between the words, all the, the meaning, buried underneath it, and then once you squeeze that down, you end up with, What we would call sort of a model.
[00:08:04] and that model is what, you know, what these large companies are selling out into the world. Like, hey, you can use this model. It represents this massive corpus of text and it can speak to and convey a lot of that. Intellectual capacity, almost like a human. so the tools that you see today, the chatbots, all these sort of new things that are popping up are really all just light, light layers and interfaces a sort of website or, you know, an app on your phone, that is built on top of that large model, which has all that sort of knowledge squeezed, baked into it. I like to think of it sort of like a, like a, Jawbreaker, it's like lots of tiny layers of dense candy squeezed down, into this one hard, big thing, that's, that's the analogy that works for me, at least.
[00:08:48] Marc Beckman: So, when you talk about these large language models, can you give example, like brand, just highlight a few brands that the audience might be familiar with or, or they might want to check out?
[00:08:58] James: sure. so the one that I think has gotten really, taken the world by storm was the GPT model. so GPT was, so it had, you know, 1 and 2 came out a while ago, produced by OpenAI, the non profit. heavily sponsored by Microsoft, you know, and they, they're now tightly coupled. but GPT was the sort of core model.
[00:09:19] What people then experienced was again, that interface around GPT, chat GPT. so chat GPT is not specifically the models per se. it's sort of the, the, the wrapper around it. but the core model, GPT then GPT 4. so those are the big ones. Google has their set. it was originally called, you know, they had Palm and Bard, and now I think they've rebranded Bard as Gemini, so they are, you know, also in this game producing very complex, very, you know, big, big models with lots of training data.
[00:09:49] Marc Beckman: So when you talk about training data, are they, In your opinion, pretty much trained with the same data, the same way? Like, are there nuances with regards to what they're, they're, essentially training, what kind of, what this corpus, as you use this word, corpus will look like, might one have more of a, background as it relates to the history of rock and roll, whereas the other is really strong with regards to recipes and cooking or like, how can we, how do we know, like, are they trained effectively the same way?
[00:10:22] James: yes and no. So, I think we These companies are working with sort of these third party providers to scrape a lot of information, but they also have sort of their own information, which they've scraped over many years. You can imagine if Google is, for example, building a model, they have tons of data that they have, scraped through their own sort of search engine processes, so they, they can draw on that.
[00:10:43] so there is a little bit of sort of proprietary information that these companies have collected over a long time, which is why companies like Microsoft and Google, they have the compute, but they also have the data from building their search engines, Bing and Google search, draw on. We are, I think, are at a limit where, humanity has only produced so much writing that is worthwhile.
[00:11:05] so you can imagine, you know, if, 70 percent of sort of the writings that humanity has produced, to train a model of these size, these sizes and this quality, No matter what 70 percent you, you pick, you're always going to overlap, you know, at least some amount. so, I suspect that these models are largely trained on very similar, if not the same data.
[00:11:26] but what makes them different is the specifics of how they squeeze that, that data down, because a lot of the specifics of the, of the text gets lost in that training process, but what you choose to remember, what you say, hey, it's this relationship in this way, I care, you know, care about this part of human understanding and not that part, that is where these models get to start to get smarter, And you can see this in examples, like, I think there was a model produced a while ago, maybe three or four years ago, which was trained on online data, and no one, I won't name the company, but no, no one wanted to put a, a, a filter on it, and it's like, hey, we're just gonna, you know, train it, and they put it out there, and then very quickly it was discovered that it was, deeply racist and sexist.
[00:12:12] robot. and it was saying pretty terrible things, and they quickly shut it down. so there has been, I think, some lessons learned here that What data you choose, even if you scrape all this information, a lot of it's not great. So you still have to do a lot of tweaking. Say, okay, how do I make this robot?
[00:12:28] Yes, there are tons of racists online and they are on these communities. I want that information because I want to understand, that text, that communication. those are people. I want to understand them. But I also don't want to make my robot think that that is a good way to act. you know, my AI, excuse me.
[00:12:44] so I have to pull it back and say, Hey, you, you know this. But being racist looks like that. Don't do that. and that is where I think there's a lot of nuance between sort of the, between each of these providers, because how they're dealing with sort of that, that last layer of fine tuning, although here's how we deal with, you know, violence or threats or racism or sexism in our model.
[00:13:06] Here's how we sort and sift or, or tell our, tell our AI, hey, don't, don't do that, but that's okay. that actually has, you know, a lot of. a lot of, I think, proprietary value because how you do that impacts the use cases that those companies can sell into. because sometimes you actually do want, to have a model that can understand violence.
[00:13:25] maybe from a, from a context of, hey, I, I want to be able to understand and detect, violence or violent thoughts maybe in a suicide prevention. mechanism in a, you know, gun violence prevention mechanism and domestic violence, maybe have a sort of a chatbot trying to aid, aid, victims of domestic violence.
[00:13:42] So you can't say, hey, we're not going to talk about violence at all. So there is, again, a lot of proprietary values of how you, how you do that, that dance, as it were.
[00:13:50] Marc Beckman: so there's also a nuance there, right, where you talk about overt racism, overt sexism, but then also training these large language models on text that might, maybe were produced at a time, you know, mid century when, a person of color or a woman wasn't even able to, compete at the academic level to create some of, you know, these important documents that are now, you know, used to train the AI.
[00:14:18] So. can you elaborate a little bit as to how these entities are distinguishing and, and resolving, innate bias issues, issues where essentially the large language model is being trained with documents that are, in fact, inherently biased?
[00:14:34] James: Yeah, I, I would like to say that I, I know they're doing a great job of it. I don't, I don't actually know that. I can't say that. they are I think keeping that information, because it is pretty proprietary as part of their mostly proprietary data set, they're keeping that information pretty close hold.
[00:14:49] but I, I do that in my experiences when these models started coming out, one of the things I do first is, you know, okay, yes, I test it, do the very low hanging fruit around sort of Sexism, racism, violence. It was like, okay, like, have they cleaned this up? And almost all of them now have, have learned that lesson, but I, I follow up with more subtle questions like, okay, give me the top 10 experts in this scientific field over the last 50 years.
[00:15:13] You mean a lot, you know, top 10 experts in this scientific field or, or, you know, academic field, the last 10 years. And you do that enough, you start getting lists and what I'm looking for is, okay. How many of those lists include women? Include people of color? and You know, I find weird things where, you know, eight of the ten will be real, and the two names that are fake, that are made up, hallucinated, are the women, the sort of female, sounding names, because they don't exist. So it's interesting how, how I'm trying to like tease out the, I guess, like how they're dealing with this behind the scenes. but you speak to a sort of a bigger point that because we are dealing with a volume of data that is so large, you know, we can't, we can't really go back and recreate a whole new corpus of information.
[00:16:04] We can't, you know, as I joke, we can't create a whole new separate earth, you know, put it, put it next to us. Make it totally free of racism and sexism and bias, have it produce a whole bunch of, you know, fictional works and have its own Shakespeare and then train on that and then bring it back to our world.
[00:16:19] Say, hey, great, we've got this perfect model. the data that we have from history, it's, it's our data. and there's, there's no other history to train on. so we have to acknowledge it and build it in, and that's why I think a lot of the work has been trying to at least get rid of the overt racism, how they deal with some of the, you know, the sort of subtle contextual racism or sexism is a little more difficult, but I think it's important.
[00:16:41] I'm hoping that they are starting to add, I haven't seen it, but I'm hoping they start to add, least interrogative prompts for the user saying, Hey, you've asked this, you know, have you considered asking like, you know, this in a slightly different way, or providing two sets of answers.
[00:16:57] Here's what you asked for, user, but here's, you know, here's, here's maybe a better way to think about it. and I've given you that answer as well. and that comes to an issue of, where the companies, these big companies are really dealing with this issue of alignment. and for the audience, alignment is really a metric of success for these big models, these companies selling these models saying, Hey, the user is asking for something. Does what I give them align with that? And that sounds great. Oh, great. My model does exactly what the user asks. but what the user says in their question, and I think we as humans, like, Hey, I asked a question, but my question is maybe, ill informed. I, I didn't use the right words because I've never, you know, I've never been in that space, or I don't know anything about laws.
[00:17:39] When I ask a question of a lawyer, I sound like an idiot. we have that experience as well with AI, but the AI is trying to figure out, well, what, what is it you really want to know? So there's a little bit of a choice behind the scenes that these models have to do. It's like, I'm trying to not just answer exactly what's given, but guess at what the user wants me to say.
[00:17:58] and then that is where we get this interesting issue of, well, now the companies, these large models, they can. Get to actually influence what, you know, what that future vision of the user is. Hey, you are asking a question and unknowingly the response we're going to give because the model, it's racist or sexist, we're going to give you 10 men because Historically, those are the lists that we have pulled in from the 70s and 80s of the top researchers.
[00:18:21] It's all men. Women just weren't allowed in the field or weren't really allowed to publish. So now, it's like, okay, well, I don't want that. So now I've got this choice that I can as a, as a team push into my mouth. It's like, Hey, how much do I want to turn on that alignment of saying, does the user really want that list?
[00:18:37] Are they aware of that bias? Do I want to let them be like, tell them that there is a bias, want to give them the alternative version just to start with and say, Hey. I'm not going to tell you about that bias. Here's a new list and I'm going to purposely, you know, draw out maybe some female researchers that were unrecognized at the time or actually, you know, you know, really stars in their field.
[00:18:55] So that's the game we're playing
[00:18:57] Marc Beckman: Yeah, it sounds to me like, again, I defer to you and your expert opinion, but it sounds to me like perhaps these algorithms will be biased, you know, for, for ever, right? Like, because you're talking about a very subtle nuance here. You're saying, for example, mid century, 1950s time period, where all of these great, you know, these great research papers, these documents, they were used now by these LLMs to train AI.
[00:19:25] maybe the documents themselves aren't overtly racist or sexist, but the fact is that during that time period, because of our culture and our community, women weren't allowed to be in that position. They're innately biased and, and perhaps that's something we'll never be able to overcome as it relates to AI then?
[00:19:44] Is that fair to assume, James?
[00:19:46] James: Yeah. I, I explained this to my wife. she's an interior designer. and very rarely do our sort of worlds, worlds collide. but I was actually talking about this topic with her specifically because we were watching a great show on, I just want to say Apple TV, Lessons in Chemistry, with Brie Larson.
[00:20:03] Wonderful show based off of a wonderful book, but it details a very, you know, a brilliant chemist. a woman who ends up dealing with, you know, issues of, of sexual assault and, you know, misogyny in the workplace, and it really derails her academic career, but she's a brilliant chemist, and you're trying to, her trying to sort of like keep her pushing forward, keep trying to survive in this ecosystem where women are lab assistants.
[00:20:27] They are almost never allowed to be the actual researchers, and she can't get her name on published works that she's actually doing the research. So even that, though that is a fictional story, it is drawing from the, you know, the real stories of many women of that era. and you could just take that as an example of like, okay, well, if all I know now, is what was published.
[00:20:48] If all I'm looking at is the paper, the paper will have three names at the top. And maybe there's a reference to a lab assistant. And the bias of our society is like, oh, maybe those three names at the top weren't actually the, you know, the thought leaders of the time. It was the lab assistant, but she was a woman.
[00:21:02] So they wanted to include her, but they were never going to list her as the first author. and like that sort of bias, it's subtle, but it is everywhere throughout our history. the flip side of that is, there's some really interesting research coming out now, about how, AI, when, when you start training these newer AI models on data that was produced by an AI, It starts to go crazy.
[00:21:29] and I'll tie these together. So, there's a, there's a type of, there's a disease, or a class of disease called, prion, prion disease is basically mad cow disease, a variation of that. When an animal consumes, you know, sort of meat of its own species, humans consume, you know, human meat, cannibalism, when cow, you know, cows accidentally eat, you know, feed, proteins that they're, you know, come from cows.
[00:21:54] It starts to create, over time, this sort of, vicious cycle with their proteins, and it starts to degrade mental capacity. So mad cow disease, is, is that, right? cow accidentally ate some cow, and it went crazy, and oop, it's not healthy to eat anymore. Big scandal for a long time in the UK, we've had some scares in the US.
[00:22:13] but now there's a, an issue with prion diseases in the AI community, for how we train these AI models.
[00:22:20] They are like brains, so it's an interesting analogy because, there's I think a report now that the Of the new internet, of the new sort of text and articles, things coming out on the internet, like 50 percent has been AI generated.
[00:22:33] So, like, there's this moment in 2023 where we had everything before that is mostly human generated, and after 2023, big chunk of it, soon possibly all of it, is basically going to be AI written. But If AI can't be trained on that data because it starts to degrade, it it you know, cascades into sort of, you know, crazy, crazy outcomes, you, go okay, so everything produced after 2023 can't be trusted.
[00:22:57] So I can't train on it anymore. Humanity has stopped producing. Reliable training data. So all we have now for the rest of time that we took the snapshot is this every, you know, like from our sort of early, you know, Gutenberg printing press, you know, text, and through all the misogyny and racism of the, you know, 18th and 19th century, and then 2023, and that we get, we hit the pause button, and if we didn't resolve any of our issues, too bad, like that's, that's where the data ends.
[00:23:25] and that combination means that We will never really get to a data set that is truly, you know, beyond, you know, beyond that bias that we had in our, in our ecosystem before. we're stuck, I suspect, with the data, before 2023. and that's gonna look really weird when you're, it's, you know, 20 1.
[00:23:43] 23. And you're like, Oh, like, how do I use, how do I, how do I have an AI that is cognizant and aware of all this, you know, the data for the last hundred years. I can only look at things from 2023 and before, until we solve this sort of this, this prion disease issue. So,
[00:23:58] Marc Beckman: That's, that's a wild concept. I really haven't given much thought to that before, but it's kind of mind blowing because as we go deeper and deeper now into the next decade of content created by artificial intelligence, which we'll see, it'll be ubiquitous. It's, you know, it's, it could be kind of alarming.
[00:24:17] at a certain level. And it's like, how do we keep ourselves in check? So like, when Ewan mentioned, you created a list of perhaps like the, the top 10 experts or scientists and, they built into it two fictional names, female oriented names, that weren't actual people. That's, the, the term of art for that is, is that a hallucination?
[00:24:39] James: yes. so. I can't, I can't possibly deduce why it would, it would, it would do that, it may have been happenstance, that they ended up being the two female sounding names, female presenting, as it were, but yes, the term of art is a hallucination, and, I think the way that people can understand hallucinations, it's not like these models, these AIs are trying to really trick you.
[00:25:04] The way that these AI models are trained is not at the word or the sentence level. It's not like it remembers a whole sentence. It doesn't even remember a whole word. It remembers a syllable. So, when you start thinking about like, oh, okay, I'm, it's actually storing syllables, you know, like got my, you know, my hat here said Virginia, right?
[00:25:21] So, vir gin ya, my, you know, my, the, the tokenization, breaking that out into three tokens, is no longer going to be the word Virginia. It's going to be, you know, three separate things. and that actually reduces the combinations of things the AI needs to remember as it starts to train this data.
[00:25:36] Cause like, Hey, I'm only remembering all the syllables in English, not every word in English, which can get quite complex. So what hallucination really is, is saying, Hey, if the AI is trying to, like, I give it, you know, a starting letter or word, it's trying to predict. Not the next word, but really the next syllable.
[00:25:53] and it's, you know, it's like, there's a little bit of variation there. It's a little bit of the roll of dice. Yes, you know, 88 percent of the time, 90 percent of the time, the next syllable would be, you know, the, T H E, or whatever's to kick off a sentence. But there's a little bit of variation. technical term here for the audience.
[00:26:09] If you want to learn something, you know, stochastic, stochastic nature to this, right? It's a little bit unpredictable. it's not deterministic, so I don't know every time. So, that's what makes the AIs useful, is that a little bit of that sort of randomness worked in, makes it feel a little bit more human, because humans don't just say the exact same thing with the exact same, you know, if I ask a question, you know, if someone asks me a question, I'm going to give a slightly different answer every time.
[00:26:31] so, trying to build that in, you have to add a little bit of randomness, but you're randomly selecting a syllable. Not a word. And what you end up happening is, as we start to build things out, particularly like names or URLs, the more, more common problem, it's randomly selecting the next syllable in a URL, or a name.
[00:26:53] So, you know, Ri, you know, you know, could end up being Rihanna. it's like, oh, okay, like, that's the most common next, if I start with Ri, the name is Rihanna. Or, and starts with, you know, ends up being Rachel. both, you know, both have a different probability and the machine might just say, Hey, the first word I generated, I thought it was going to be Rachel because all the text said Rachel is the first name and ends up saying Rihanna.
[00:27:16] and it's like, okay, well, I can't go back. Now I've got to invent a name, a last name for this researcher who's Rihanna. so it's now it's Rihanna Smith or whatever. Totally doesn't make sense. Totally doesn't exist. so the AI, when it makes those sort of randomness choices, random mistakes, mid name, sometimes it has to correct and say, oh, well now I've got to guess, at a name, and the names are totally invented.
[00:27:40] And for URLs, this gets really, I think there was a lawyer who got caught on this, of citing, citing legal arguments that didn't exist, and it's, you know, a lot of people made the mistake when these models, you know, models came out, because if you imagine an AI trying to give you a URL, It might be, you know, somefutureday.
[00:27:55] com slash, you know, episode one dash, you know, James. but the way it's going to construct that, it's going to go, you know, some, even if you get somefutureday. com, right, that's pretty common, slash, episode. And then it's gonna, you know, episode 11, one, one, no, no, it's gonna be episode 11, and then dash, and it may not, no, there may not be an episode 11, so it's like, oh, dash, James.
[00:28:17] so there's only one part of that has changed. It looks and feels like a real URL, but then you click on it, it takes you to, you know, a 404, it's just like, hey, there's nothing here, that's not a real episode. so, it's that sort of stuff because it's at a syllable level that the URLs and names start to break down.
[00:28:33] and they're trying to get better at this. They're trying to solve this. but most of the tricks that I've seen to solve these issues around URLs and making real links, is to actually have a separate system that goes and finds the real URL, tells the AI, Hey, use this thing, this whole thing.
[00:28:46] You're not allowed to change it. And then it tells you, it tells you, oh, this is the URL that secretly, you know, a separate system actually got for me because I can't be trusted to create it on my own.
[00:28:56] Marc Beckman: It's kind of interesting, James, in this presents, like, this 2023 demarcation of information input and output towards our society, the way we consume content and gather the news and all, it seems like maybe there's going to be a new, cultural, philosophical, ethical, and moral, challenge for us as a society.
[00:29:19] Like, what information Is reliable. We, you know, we live in this time period where, people don't like to do the work, right? They don't really want to go in and spend time reading long form content, doing the research, checking, checking the facts and all, we'll be complacent. We'll read something in the news.
[00:29:35] We think it's from a good source. Meanwhile, that news outlet might have picked it up from, you know, a different source, which wasn't a good source, and it kind of like snowballs all over the globe. We get news that really isn't real over and over again, and we're complacent with it, so it seems to me like there might be, a new type of societal issue that we're faced with as it relates to the validation of news and, and information, we might just look at those two names on that list that you're presenting of 10 people and just say, okay, that's, those people are real.
[00:30:08] And it might just get lost in the shuffle of, you know, the news, outlets just disseminating information, or we might just take it at face value. so, so the issue of news validation, content, information validation for a society, I wonder if we're up for that challenge. I wonder if we can, you know, if we have the leaders and, and also the, fortitude to, to challenge ourselves and, and dig into that.
[00:30:32] and I think that's particularly interesting. as it relates to the fact that we're, we're, you know, election season is upon us. There are some small elections that, we just have been experiencing recently. I think recently we read about and heard that there were some, deepfake robot, calls with Joe, Joe Biden, President Biden's voice.
[00:30:54] can you talk a little bit about how artificial intelligence can take on, the sound, the voice of a person, their, their image, their likeness, and then, what kind of problems, and perhaps what benefits also that could create, for
[00:31:09] society.
[00:31:09] James: I'll be honest, I haven't actually thought about the benefits yet. so that, that's, that's not where my head space is in. but, yeah, we,
[00:31:16] Marc Beckman: just, just to throw it at you, you know, here in New York City, Mayor Adams, was heavily scrutinized because he used artificial intelligence to put a message out to his constituency in multiple languages, and, obviously English is his first language, and in his voice, but speaking Spanish through the, tool of artificial intelligence, he gave the same message to, you know, New Yorkers who, prefer to speak in Spanish and he was, he was highly scrutinized by that for that.
[00:31:45] So like, you know, there's an issue. Is that a benefit to the society? They're getting a clear message in the language that they understand. It happens to be in the voice of the mayor. So it's people knew it wasn't real. so perhaps it's beneficial, but you know, let's start off with the downside because certainly it's more prevalent, risk
[00:32:03] involve
[00:32:04] James: Yeah. So, I think was it Mark Twain said it was like a lie can travel halfway around the world before the truth gets its boots on. so I think that. You know, Mark Twain was not alive when we had AI, pre, you know, it's pre computer, it's pre, pre vacuum tubes, so I think that society's always had an issue with dealing with sort of truth and media and scale, and I think that, that has been a struggle we've had for a long time.
[00:32:32] I think AI is really just changing the, Not the nature of that, really the price point of entry, you know, the how much does it cost to get a lie out there quickly, and effectively, I think AI is really just bringing the price point for the players in that space down, for better or for worse, on the sort of the AI side, I, you know, I was really enthused, you know, excited to see that the FCC sort of very quickly responded and said, hey, we're There's a rule now, you cannot use, you know, electoral, you know, you cannot do robocalls with people's voices without letting people, letting people know, one, without permission of the person, and two, without, letting people know that it's an AI, AI generated voice.
[00:33:12] and I do think that that is a good first start, because it is, it is approaching the issue from the, sort of the reality is that the reason that lies are effective, is not so much that the lie is, You know, it's not so much that the lie is told to you a lot. It's more that the lie is subtly, it sounds right.
[00:33:34] It plays on those biases. I'm going to circle back to sort of scam emails. actually, let's just, let's just begin. so if you are designing, for example, the Nigerian Prince scam email,
[00:33:47] Marc Beckman: Yeah. I know that guy.
[00:33:48] James: follow me down this rabbit hole. if you're, if you're designing, if you're a scammer, and you're like, and scamming is a business, right?
[00:33:54] There are, there are office buildings in, you know, Eastern Europe where people just do this. This is their, this is their job. so if you are a scammer, and you want to convince people, it's like, hey, give me money, you actually don't, you know, the game theory is you don't actually want a lot of people to respond.
[00:34:10] You, so you, you want the people who are the most gullible to respond because I can't sift through a thousand emails. I want to, I want to go to the two people that I'm gonna get the, the quick, the quickest amount of money from. So you design the email and you can see this is like, I designed the email to have this big grand number.
[00:34:26] But it's full of grammar mistakes and it's like a weird sounding email. you know, most people would read that like, oh, this is, this is total nonsense. But they're actually, you know, the scammers actually leaving clues in there so that the hyper, you know, aware and rational people don't respond because they don't want to deal with them.
[00:34:42] they want the super gullible. and I think like what we are dealing with now, is the, if, can AI change that calculus? Like, hey, if I could just have an AI, right. All the emails and they can be specific to that person. They can sound so natural. Now it's only on the far side.
[00:35:00] It's not, it's not detectable. It sounds reasonable. they, oh, they use a friend's name that, you know, was scraped from some, you know, Facebook or LinkedIn connection or data leak or whatever it may be. It's like, hey, blah, blah, blah. I need this. Here's a link. that is a much more terrifying prospect. And again, that used to be, oh, I had to, I had to write those 10 emails to convince that person.
[00:35:19] I do them. And if you're dealing with people in in, in sort of Eastern Europe or any other parts of the world where English isn't their first language, that's going to be hard for them to sound like corporate and savvy, but an AI can absolutely do that. So you've got this sort of big shift in the price point for a very convincing scam email.
[00:35:37] And you can see this in a lot of the phishing emails and whatnot. And that whole, that whole ecosystem just with scam emails and phishing represents the broader ecosystem problem with these sort of very good AIs. You've got now phone call, you can do with phone calls, you can do with video soon.
[00:35:54] And this idea that If I can't trust, even as a well informed, you know, educated person, if I can't trust what I'm seeing, how do I then interact with society? How do I vote? How do I, how do I engage? How do I, you know, donate to causes, if, you know, if I don't really know if the cause is real, if the person on the phone is real?
[00:36:16] and we've, you know, we've always been do those lies. We've just made the lies now way more Way cheaper to do very effectively. and, you know, we can all say that, hey, like, people shouldn't do that. but there's always going to be bad actors. And even if the FCC outlaws it, there's still going to be people doing it.
[00:36:34] and I think we as a society have to feel out how we want to deal with that trust issue. And I think that speaks to your, your story about some of the New York mayor. While on its face, it sounds great. Hey, it's a trusted, it's a trusted voice. And it's in the language I speak. Those are net positives.
[00:36:51] But he, I think he tapped into something that we haven't dealt with yet. It's like, Oh, but like, I know he doesn't speak my language. And now, now my guard is up. Oh, do I trust him? Is this, is this real? And now I'm panicked. Now I'm someone who's like, I'm getting calls from a fake mayor telling about something.
[00:37:05] Oh God, do I trust it?, So he, I think inadvertently tapped into a panic, you know, hit the panic button in people of a trust issue, even though he was trying to do something. Right. And people, people were upset. I would be upset by that. I was like, ah, this, this, it feels weird. I don't know how to deal with that trust because now I'm being asked to judge something for its trustworthiness and its value.
[00:37:27] And I have, at this point in my life, very little experience, and I have probably more experience than most, but still very little experience, you know, given my life. Like, oh, that's AI, and I know that, and here's, here's like mentally the bucket I put it in when I consider like how much credence to give it.
[00:37:41] Marc Beckman: And it's, it's hard to distinguish now with regards to the image of the individual, whether it's a still photograph or a video, the sound of their voice, their voice itself. I understand that there was a recent scam using deepfakes in Hong Kong just this month, in fact, whereby an individual was duped out of handing over about 200 million worth of cryptocurrency as a result of having, it's my understanding, a Zoom meeting with five or six of his or her colleagues and each person on that Zoom was a deepfake and they actually handed over The Cryptocurrency as, as a result of that, it's interesting if you go down a little deeper in this rabbit hole of, you know, nefarious behavior, people are talking about Elon Musk's, one of his innovations, Neuralink, which is essentially their, their brain computer interface.
[00:38:44] And I realize that at the core, it's not about artificial intelligence, but perhaps you can explain a little bit, what Neuralink is, and then. What happens if that bad actor wants to use artificial intelligence across the people connected with Neuralink?
[00:39:00] James: So, That's sci follow up on that Hong Kong story, I love it because I think it really paints a picture for what I would say is like what people should be aware of, you know, audience members out there listening, it's like, oh, like, well, How should I re change my thought process around being tricked, scams?
[00:39:19] because I think it used to be, hey, it's going to come in an email, and it's, I'm going to be interacting with one person, the scammer. But that is no longer the case. You could actively be on a Zoom call with seven deepfakes, where it's, you know, seven different people, All, you know, talking as if they're real, but the voice sounds real, the image sounds real, and oh, the connection's a little blurry, the camera's a little blurry.
[00:39:40] I don't think the average person would say, hey, yeah, seven people are gonna spend an hour on a Zoom call with me having a corporate meeting to steal money from me. that was just, it's absurd. but I think, you know, heading into election season, my sort of nightmare scenario is, okay, well, that's, that's expensive to do, but that dude had, you know, millions in crypto.
[00:40:01] If I'm looking for someone to vote. I don't have to have one person. I could say, make, you know, a new echo chamber in social media, say, hey, you know, the nightmare scenario is like, hey, my parents are trying to understand a new topic, something emerging in technology that's scary, like AI, and they go online and they start interacting with it, and there are ten people in a chat room or on some message board, and nine of them are bots.
[00:40:25] And they are the 10th. And they would never know. And that, like, that is, that sort of dystopian to me is like, you'll never win an argument with a bot. You'll certainly never win it with nine. It is a war of attrition. They will just slowly push back. Slowly, you know, one will be aggressive and one will side with you, but then you know, walk you forward.
[00:40:43] Like, it, You can do a lot to manipulate people, particularly when they don't know they're being manipulated, and you, you crowd them out. and we are, we are absolutely at that point, in our technology, and the Zoom, I think the Zoom meeting is just like, takes that example to an absurd level, but it happened, so we're gonna have to deal with that.
[00:41:01] on the Neuralink side, I can't say I am a, you know, biologist or, you know, a doctor in the space, but I do think. that is interesting, there are a lot of ethical issues with how Neuralink has gotten to the point where it's at. I think, you know, maybe some, you know, some ethics review boards might have been, might have been, you know, necessary.
[00:41:22] but Elon Musk is pushing boundaries. so the Neuralink for the audience, as I understand it, sorry, he's putting, called a, a small array of sensors in your brain. it's like a chip. In your brain, with some wires that go around the brain, typically you see this in sort of people, you know, when you're watching movies with people, all those little electrodes in their head, they're doing brain studies, typically those things are done on the outside of your brain, which is fine if you're just trying to do a sort of a passive, a passive interaction, but it's also, you know, it's, it's like trying to, trying to hear, you know, play a game of telephone, With really complex, you know, really complex sentence.
[00:41:59] Someone's trying to tell you the sentence, but they're holding a pillow to their mouth. so, you know, try trying to talk to your brain and read those sort of, electrical pathways at a fine grain, you know, fine granularity through your skull. And hair is hard. so a lot of the research has actually been done, on people who have had to have those inserts done in the brain for other reasons.
[00:42:19] So hey, I am an epileptic and I've surgically had to have these implants done. And then they ask those epileptic patients to volunteer for other studies around these sort of neural linkages. it's sort of like, hey, you've already, you've already got the hardware, come, come do some research. And I think, as I understand sort of Elon Musk is trying to.
[00:42:37] Commodify, that technology might be the way. It's like, okay, well, how do I, how do I make it easy? you know, you can think of the, you know, people got all those, like, I used to have to go to an orthodontist to get braces, and now I can get some of the, you know, Invisalign. It's like, hey, everyone can get these little plastic things.
[00:42:51] It's a medical device, but it's, it's quick. It's easy to show up at our clinic, and we mail it to you two weeks later. I think Elon Musk is actually trying to head towards that. I'm not sure what the output is, what the benefit is, because I don't think we've seen sort of like, hey, I can't, like, Read a computer, read digital information with my brain, but we don't know.
[00:43:11] The first step, however, is a large dataset of people with these, you know, Very, know, embedded implants, not, not the sort of outside the skull. And there are some advances on the outside the skull. There's, I think, a, programmer who, was playing Call of Duty with, like, with, like, with her head and, just like a, like a, a passive receiver, and she was just, like, thinking moves and like, nodding her head or moving her eyes, like, very minimal movements of, like, if someone's trapped in a wheelchair, like, could this work?
[00:43:40] And yeah, she was playing Call of Duty and, like, you know, actually killing, killing other players. It was, it was really
[00:43:44] Marc Beckman: I think that's the essence of it, right? It's to commodify it, but it's also, it allows for you to control a computer or to control a mobile device with your, with your brain, right?
[00:43:55] James: right. And, you know, there is already that research being done, in the medical community by medical experts with people who are, you know, not fully aware of the risks of that sort of procedure and are probably, usually, because the risk was so great relative to the benefit, they're only doing a sort of, hey, I've already got this disease, I already need the hardware.
[00:44:13] For a medical issue, but yes, now you can do research. And I think trying to, trying to flip that to me is what worries me about Neuralink is it's pretending to be a value proposition that isn't really there yet. It's like, Hey, do this thing. And it'll be great. I don't know how it'll be great. I don't have data to prove it'll be great to get that data.
[00:44:31] I have to test on you specifically. That, that sort of ethical balance of like surgery, heavy surgery on your brain. And like a potential benefit is I can move a mouse with my, my, my, my mind seems like an imbalanced, but he's he's had it you know he's heading into that. Yeah,
[00:44:49] Marc Beckman: interesting, though, because it could, I think deepen the digital divide between the haves and the have nots, too. you could imagine that eventually if Neuralink is, you know, a fantastic technology and it's, not as invasive as it sounds, it sounds actually kind of horrific, frankly speaking, but if it's, you know, seamless integration into your body, A person with, the benefit of Neuralink could be sitting in a board meeting, with some peers that don't have access to a computer and in real time answer the questions.
[00:45:21] They could be looking to get a job that's worth a lot money, a lot of money, and in an interview have access to that data and that information. I guess the scary part of it beyond the, the obvious, the, the, you know, the physical part of it is, if artificial intelligence is, being used in a way that is nefarious, whether it's wrong messaging or, or a deep fake, a lot of people can end up having this information vis a vis Neuralink, I guess, put into their mind, but so, you know, it's an interesting place we're going and it, you know, lends to the idea of, you know, whether or not we're going too fast with regards to building new technologies and artificial intelligence in, in particular.
[00:46:08] Right now, effective accelerationism is a big topic in, in the tech community. I think there's. Part of the argument or a strong part of the IAC argument is we need to accelerate to save humankind. And I'm wondering, in your opinion, should we lean into it and continue to accelerate this quickly? Or are we risking a situation with artificial general intelligence where, you know, we're going to actually kill.
[00:46:38] Humankind.
[00:46:39] James: yeah. And I do want I do want to clarify to the audience right now, like, as much as the on the Neuralink, as much as we talked about it, it is an out is an outbound messaging, my brain can tell the chip, something, the chip cannot tell my brain something we are far from that. So, if you think like, oh, I'm gonna become Neo in the Matrix and get plugged in and, you know, have all of Wikipedia at my fingertips, we're very far from that, but, you know, too bad, that'd be awesome, I would love to know Kung Fu, but I, you know, but the ability to Control devices, and outbound control that is possible.
[00:47:21] but I am more excited by the AR and VR augmented reality. So systems that get a little bit more fashion friendly. so instead of the, you know, the big heavy vision pros that Apple puts out, but like more like the. Facebook and Ray Ban partnership, like, hey, I've got these glasses, which have microphones or headphones built into those jawbone, you know, skull, skull things where you, there's no speaker, it's just resonating with your head, and it's got a, you know, an AR display in the glasses, but it looks fashion forward.
[00:47:48] I think that sort of interface of receiving information and engaging, is going to be much, much more common in the next 20 years. And that's the direction we're headed. and I think Neuralink is going to end up being more of a medical thing for a while. but the, the ability to write electrodes into the brain to influence is, we're far from that.
[00:48:10] Even chemically speaking, antidepressants are mostly just like Chemical soup. We just throw it into the brain and in a big soup. It's not targeted to specific, specific regions or problems. It's, it's soup.
[00:48:22] Marc Beckman: so
[00:48:22] you think the first step then is like MR, AR, VR, Apple's Vision Pro, you think is a, is a big step forward in that direction?
[00:48:30] James: Yeah, what, what I liked about Apple's VR Pro is that they upped the game in terms of the sort of the quality to a sort of consumer threshold. I mean, I love, you know, I think Oculus was great. I think, you know You know, Facebook Meta, you know, is an interesting play to push for the Metaverse.
[00:48:45] I think maybe they're a little bit too far over their skis on that one, but it is coming. I think their partnership with Ray Ban is a much better signal. Because like, hey, Ray Ban is a known brand. It's fashion forward. And if you want to start having people really use this, you can't be something big and heavy and clunky.
[00:49:02] That's the big complaint with Apple Vision Pro right now. It's got to be more like, oh, I've got glasses. I think we're headed towards that, and just like the, you know, I think the analog of Apple's iPhone, early first iPhone, like, it couldn't copy and paste for two years, it seems silly now, but that, that was the, you know, that was the use case at the time.
[00:49:20] It got better. It got way better. even as bad as it was the first round, and Apple is a company that, You know, like, yes, they have some failures, but they know how to make things better if there's, if there's viability. So, I do think we are headed toward the AR VR space, and that that will crowd out any benefit of Neuralink, because if I can get 90 percent of that value as a consumer, and I don't have to have major surgery, I'm probably gonna do that.
[00:49:43] so, so yeah, sorry, what the, I, I did, we circled back on that, we were talking about, oh yeah, accelerationism. I I like the idea of accelerationism in some contexts, climate change, for example, I think accelerationism is, the right path forward, but it's the right path forward because, because of the governmental construct that we're operating in, right?
[00:50:09] The world has not figured out how to get together and say, hey, stop producing so much fossil fuels, let's all collaborate. we keep, we collaborate enough to talk about it, we're not collaborating enough to really do anything. Like, we're doing something, but it's not nearly enough. it's not like we're producing less carbon, we're just producing more at a slower rate.
[00:50:29] So, you know, we're, we're still, we're still heading towards disaster, but a little bit slower. it's not a great sign for us as a, you know, our ability to operate collectively. so I, I think My bet is, in fact, on technology in that space, I think that even like the patents that are coming out of Shell and BP and, you know, battery technologies, the large energy companies, they are, while they are fossil fuel companies, they are hedging their bets and they're investing in non fossil fuel energy systems, they They wanna be energy providers writ large and own that transition.
[00:51:03] and I think helping them, you know, tax breaks, whatever, encouraging them to say Yes, go invest more, invest faster in this space, is gonna be, better. The fact that we've got, you know, all these electric car, you know, grids, whether you like Tesla or not, the fact that they had some sort of charging grade set up.
[00:51:21] Superchargers, it was a big step forward and it really did change the car industry.
[00:51:25] Marc Beckman: It's incredible.
[00:51:26] James: It didn't quite have the effect that we wanted because the sort of the environmental impact of charging a car and producing that electricity is still worse. you know, running these AI fancy cars is still worse than a combustion engine in terms of total energy cost.
[00:51:40] and a lot of that electricity is still produced with carbon. So, you know, there's, but we're getting some of the pieces, the pieces in place. so in that context, climate change, geoengineering, I mean, even China's starting to modify weather, like, at some point I think we're gonna We're gonna, the crisis and the weather events will get so terrifying and bad that countries, maybe not the US, but someone, you know, you can imagine Indonesia, India, China, who are gonna be much more dramatically impacted sooner by climate change and rising tides and bad weather, that they're gonna act on their own.
[00:52:13] Say, hey, I don't care what you guys think. I'm going to make the, I'm going to start cloud seeding. I'm going to start reflecting the sun. I can't deal with this. so I think there is that sort of potential unilateral action path, which, you know, for better or for worse, like it's like, again, it's nothing to do with US policy.
[00:52:30] It's just. What would I, what would I bet that someone else is going to do it? and acceleration in that, that sense, great. I'd rather, I'd rather that technology be good and faster and here, and everyone actually work on it together, then pretend like we're going to survive. On the AI side, I have the opposite.
[00:52:48] the AI. AIs, the issues with AI are, it's not like acceleration is going to solve a governmental problem, issues with AI are actually going to exacerbate the government problem, governance problem, if you think the biggest threat from AI is, the loss of jobs. I think that is a, everyone understand that.
[00:53:10] Oh, I had this knowledge worker job. Oh, I was an artist. And now, you know, now I, I, an AI can do my, my work better than me. the joke, you know, I, I, the joke I make is that, you know, this is, it's even coming for comedians, right? We are not far from a future where stand up comedians are not as funny as the AI writing their jokes.
[00:53:29] and it's like, what's the point of being a stand up comedian, if an AI is funnier than you? so, you know, all knowledge jobs are, are suspect. a lot of creative jobs are subject, music can be written by AI and sound like the artist. so if that is the context we're heading to where the knowledge worker jobs, particularly the ones that the U.
[00:53:48] S. has been this is our economy, based on, sort of knowledge workers, if those go away, our economic advantage goes away. And we go back into a very fast era of re, you know, renewed globalization. And for those who had jobs in the, you know, the 90s, in the early 2000s, when the internet, you know, even late 80s, when the internet was blowing up and jobs were getting offshored because they could, technology enabled offshoring.
[00:54:14] It didn't need to be physically present to do a lot of this stuff. that offshoring, like, really disrupted industries in bad ways. And the problem wasn't wasn't that these AI tools were, or these internet tools were bad, it was that the pace of change was faster than society's ability to deal with the loss of, of income for all these people.
[00:54:36] It's not like I'm a, you know, I'm a, you know, a mill worker and I can suddenly learn the internet and AI. It's not like, oh, I'm, I, you know, spent, you know, 20 years becoming a really, really good accountant and now an AI can do my job. I'm not going to then go, you know, oh, I'm, I'm going to go be a barista.
[00:54:51] Many people did end up having to do that, and there, you know, that's a massive loss of income, and our society wasn't really so structured well for that, and a lot of people lost out, and we wave our flags like, hey, free market, this is better. Yes, we can say, hey, we're headed towards that, we do want to do that, but how do we do that in a way that is maybe a little less dramatically dangerous for our society?
[00:55:13] It drives fewer people into poverty, it maintains, you know, revenue for school systems, and all these things we have structured into our, our system. We cannot have a capitalist system that says, hey, Your, your ability to like to feed yourself is dependent on your job and then be like, oh yeah, now we're going to release the technology that just destroys all these jobs and pretend like the two aren't related.
[00:55:35] you know, we have to balance both. so I think there's the, that accelerationism in AI, it is coming and I think we need to maybe temper it. Let's learn the lesson from the 90s and say, hey, let's just go a little slower. and think, think a little bit more cautiously about this. and the.
[00:55:51] The example I bring is, I think you and I were talking about this, before, people, the term for people who hate technology is Luddite, and I love this, like, people pretend, like, oh, accelerationism is this brand new thing, oh, just, like, lean in the future. I don't think, you know, the, the Peter Thiel, whoever it is, I don't think they actually know what the future is.
[00:56:12] They, they, they're promising great things, but they don't know. They're betting. and he's a billionaire. He's like, he's gonna be fine regardless. the rest of us, I don't know about. so I would give that a little accelerationism. You know, they're betting on an unknown. and they're gonna make out like bandits.
[00:56:26] We may not, so I'm a little scared in that respect. They pretend like, oh, it's this brand new thing. Of course, it'll be better. But if we look at All the history of humanity. Actually, we've done this a few times and it's failed, in the 90s, but the original one, was actually the story of the Luddites.
[00:56:43] And we, the term is now, like, you know, talk about sort of technology winning over. We refer to people who hate technology as Luddites, but that actually wasn't the Luddite movement originally. They were, I think, wool weavers, loomers, right? And then the mechanical automated looms came out in England and we're like, Oh my God.
[00:57:01] My whole business, my livelihood, my family, my children will starve if this, if these things go into production. And they said, hey, we, we know this is coming, but can you give us a little, like, can you give us a little bit of a social safety net? and this is pre, you know, pre the sort of, post war socialism of the UK.
[00:57:18] This is a cutthroat capitalism UK. so they, you know, so they're, they're protesting, hey, we, we need a little bit of, you know, help here to sort of transition. And the, you know, parliament at the time was like, nope, best of luck, harsh, you know, harsh free market capitalism. so these people went from like having a job and having, being able to feed their children to suddenly, you know, absolutely impoverished.
[00:57:41] And it was a whole industry and whole, these were parts in areas where like towns were built around an industry. So you had whole towns overnight, suddenly impoverished. And those people got upset. And then they set fire to, to the factories or the looms. Maybe not the best idea, but I can understand like, hey, like.
[00:57:58] My children can starve or, you know, I'm setting fire to this thing. The role of government in that, I think the role of government in that, that, that space was like, this is where government failed to deal with that transition. It's like, Hey, we didn't need to burn down the factories. We could have actually found a soft landing, for, for this group.
[00:58:16] and still advanced our technology in our industry. and they pretended like, oh no, we're gonna ignore it. And of course that led to violence. and you know, and deaths, I believe. So, we have failed at this many times before. and putting our blinders on to accelerationism, is, and the cost of that is where we fail.
[00:58:35] But I do think the technology will come. We are, we are, we are moving forward. How fast we go. And how deal with the consequences of that speed is the, is the question that I hope people start to think about.
[00:58:48] Marc Beckman: just to, just to play devil's advocate with you for a second, is it practical to believe that, commercially our, businesses here in the United States will slow down, so that they could be more prudent in the way that you're talking about? And then on top of that, what happens with regards to influence?
[00:59:11] as you had said earlier in our discussion, effectively, the way that these LLMs are trained, become knowledge sharing centers. So if we're not as a nation moving forward and, putting the foot down, the gas pedal down as it relates to innovation, capitalism specifically for AI, will that hurt us?
[00:59:35] Is it practical? And then also, will there be LLMs perhaps someplace else in the world that will have a different type of information sharing system that doesn't align maybe with American values or Americans take on history? Who knows if it's right, but you know, these are, are issues that I think we would be looking at if, if we slow down.
[00:59:56] Is that, is that a concern of yours?
[00:59:58] James: yes, and I think I would counter is that I'm not suggesting that we slow down. I'm suggesting that we acknowledge the cost of speed and saying, hey, you know, it's like, hey, it wasn't like, hey, don't build the looms. you know, let the Luddites be for a couple years, and then we'll slowly, you know, build looms.
[01:00:18] He's like, no, build the looms, and then help the Luddites, you know, transition to new paths. Maybe, prepare, you know, like, and like, we tried this a little bit in the 90s with drop job training. but it doesn't really work, right? It does, like, you can't be, you know, like, you know, I guess like a 20 year accountant and then suddenly learn, you know, like, Oh, I'm going to go to the mines, like
[01:00:38] Marc Beckman: gonna drop out. Yeah,
[01:00:40] James: right.
[01:00:40] So we, you know, this also was in the pandemic, like there's this, you know, some people just couldn't deal with the transition to remote work, and didn't want to, so dropping out of the workforce, when you are able bodied is a drag on the economy.
[01:00:53] So like even the. you know, small, C, conservative, most fiscally conservative person in our country say hey, we actually want better, greater GDP, we want people in our workforce, we want people with knowledge and skills to actually be engaged, and spending money and making money and spending money.
[01:01:10] that is how our country stays ahead. so, if we are going to say, yes, we, you know, if, trying to regulate and slow down industry, I think you pointed out, it's near impossible. you can, you know, put a little, little sand in the gears there, but not too much. You can't stop it. I think you could look at the history of cryptography and internet security as like, nope, like, it's gonna, it's gonna happen.
[01:01:32] The technology's out there, it's gonna happen. but we can at least maybe offset that risk, with you know, Put, you know, put my, more progressive hat on, but this idea of, oh, well, maybe a little bit more of a social safety net for people impacted by this specific thing. and maybe AI is actually the solution to that.
[01:01:51] Think about scaling and job programs and retraining and opportunities. mean, a lot of people in the, in the, in the last, you know, financial crisis, whatever, you know, 2008, a lot of people lost jobs and like, they didn't return to the original job. A lot of them, like, and, you know, the era of, I think, gig working blew up in that ecosystem of like, oh. It's like, oh, like, I, I can't get a full time job anymore because my industry doesn't exist. I guess I'll just drive my car around, and get paid by the hour. so like it is, or by the, by the ride, probably not what people imagined when they got their degree and spent five years building their careers.
[01:02:23] Like, oh yeah, I, I want to be an Uber driver. so I, how do we tap into people's excitement and potential and their existing experience in education in a way that is thoughtful and fruitful for society? I don't know, but I do think that. If, if we're gonna start cutting jobs, we need to find ways to create more jobs for that audience that is not as far a leap from their existing skill set.
[01:02:50] you know, don't ask the, you know, don't ask the accountant to become a barista, if, if you want him to be happy. Because he may be like, nope, I'd rather just retire.
[01:02:58] Marc Beckman: But we've, we've seen, like, historically with the advent of new technologies, we've seen job growth, we've seen job creation, new industries, new needs, as new demands come out of these innovations. I mean, certainly we saw it as it relates to the automotive industry when we moved from, you know, I, I remember, reading recently a, a story here in New York City, people were up in arms because, the, the addition of cars on the road, we're going to kill people.
[01:03:26] The very important and very popular hay industry that certainly that was all around New York City. I don't know if you're aware of this, but like people were really up in arms because of it. But sure enough, with automotive, more jobs came, different types of jobs that people didn't even imagine. And, you know, in a way it, you know, certainly had a positive impact on GDP.
[01:03:47] And, I think it also helped rise the quality of living, the standard of living on a, on a local scale, for sure. In some ways, arguably, but, you know, access to better quality healthcare, better quality food, et cetera. I think if we, if we can, find ways to leverage this new technology, it could be very beneficial.
[01:04:06] You mentioned education. I think it's, interesting to look at artificial impact, artificial intelligence impact on a global scale. I think there's going to be a certain level of rebalancing worldwide, right? Like, no longer. Arguably, no longer does the individual who's working perhaps on a farm in Sri Lanka that wouldn't be able to access westernized university, Oxford and Harvard and even New York University, because it's very expensive to, to, to, you know, attend these universities.
[01:04:37] Well, now they could certainly just access their phone. Everybody has a mobile phone and receive education from artificial intelligence on a particular topic. And, you know, take that concept a little further, maybe even be innovative and creative and start a business of her own or his own. how do you see artificial intelligence impacting, I think the education structures that we have, the traditional Westernized university education structures that we have, do you think there'll be an international rebalancing?
[01:05:08] James: yeah, on the, on the horse, horse analogy, it's not only, not, I love this one, it's not only the hay industry, it was the manure industry, which actually had a, because I think New York was producing 100, 100, 000 tons of horse poop a day, in New York. So, like, the, the, the carts to move manure from the city to the farms.
[01:05:33] was massive. It was like a huge industry. that, and that was, that was the one that actually, because it wasn't like, it was farms depended on, on that manure. but I, I will also argue that like that transition happened in an era when the U. S. was very more ruthless, ruthless capitalist, you know, free market.
[01:05:52] It's like, we, we didn't have a lot of job training programs and like, we could say, hey, yeah, we've got cars now. Yeah, cars survived. The technology won out. I'm not, I don't know if the people who had livelihoods and, you know, like at the time that were, you know, ruined, was, were so happy. But I also know that the speed with which the car took over was slow.
[01:06:12] It wasn't an overnight thing like AI. It was like a, oh, like, because you have to manufacture cars, you have to train people, like, only the wealthy could afford them. So it was a while, but from like the early car until like, you know, the, the Model T with Henry Ford, and even then. You know, the price point had to come down, so there was, I think, a transition of at least one generation of the people who grew up the son of, I'm the son of a, of a, a, a hay, a hay salesman, a maneuver, maneuver, like, oh, I can, I can, I don't, I, that, that job's dying out, I'm going to go get another one.
[01:06:42] There was a generational gap, and, and that one generation gap is, is what's really required in these transitions.
[01:06:48] Marc Beckman: It's a fascinating story. It's not told enough with, in my opinion, as far as like, history of America, as well as how it relates to renew the history of renewable energy. That first, those first cars that hit the streets in America were actually powered as you're, I see, you're aware. they were, they were electric powered.
[01:07:07] They were battery powered, and then. We moved into fossil fuels, gasoline, oil, later on. And it's funny how things come full circle. Now we're talking about Elon and Tesla and everything, but, but you know, that we're going back to where we started. We're going back, you know, it's back to the future, right?
[01:07:23] James: Yeah. Yeah. Back to the future. Yes. so on, on the ed tech side, education side, so this is a, a passion space of mine. so my parents are both teachers, high school teachers, and I, worked in education AI space before this most recent wave of AI. and you know, begrudgingly my investors said, I don't think online education or remote education is ever going to be a thing.
[01:07:45] Shut down that company like a couple months before the pandemic, because. I couldn't convince investors that online education was really going to take off, and AI, AI based education was, was coming. So, while, while I am, I think I have good foresight, apparently I'm not a, not a great salesman.
[01:08:02] lessons learned there. but on the, I mean, I absolutely do think that AI is going to shape the nature of education. I think that We've tried to do similar things in these fits and starts. There's a movement in the education space, the flipped classroom model. And basically for those unfamiliar with it, the idea was, teachers, like the sort of typical style of teaching for a long time was a, the teacher is this bucket of knowledge.
[01:08:29] Kids all have heads that are empty buckets and you pour a little bit. The teacher just pours a little bit in everybody's head, right? It's this dump of knowledge. And I'm sure a lot of people you know, our age, or, or, or older remember, like, oh, you're sitting in classrooms and the teacher writes a whole bunch of stuff on the board and you just write it down.
[01:08:47] and like that was, that was school. that, that structure is pretty terrible, pedagogically speaking. It doesn't lead to the best outcomes. so there has been sort of movement. It's like, oh, how do you make education more engaging? How do you make teachers more responsive to students needs? And.
[01:09:03] What online video is, so, so, you know, early, you know, early sort of 2010s online video comes out and says, hey, If all we're doing is standing up in front, if all the teachers are doing is standing up in front of a class and teaching kids, like, it's just one way dump of information, we could do that on video.
[01:09:19] And, if, you know, like, if we've got time with kids, that's precious. So, let's record the videos, make watching the videos the homework. Go home, watch the lectures. Then come to class, and we're just going to do all the interactive stuff. We're going to do the homework together and in small groups. So the engaging part, the hard part of like, oh, I've got to challenge myself, I've got to try.
[01:09:39] It's a lot easier because the teacher's there to help you. Like, oh, I don't get it. As opposed to just dump information, then you go home and you're stuck trying to do it by yourself.
[01:09:46] Marc Beckman: so effectively more immersive and collaborative versus being spoken at, spoken to,
[01:09:51] James: Yes. And you can also see like how a lot of the educational outcomes that, you know, we are dealing with today are based off of home life. And part of that is because The formative assessment is like, think of it as like tests or quizzes that you take at home, not to get a grade, but to learn, right?
[01:10:09] It's the thing you struggle at so that you can actually learn and then take the summative assessment at the end. Like, hey, I proved that I learned it. So homework is basically just formative assessment. But the success at homework, homework is this vehicle of learning. It really depends on a couple things.
[01:10:23] Like, do you have a house or home life that enables you to do homework? Are your parents educated enough that they could help you with the homework? Because. Are they even around? The only people there to help you at night with homework are the people in your house. Otherwise, you have to have, you know, afford a tutor, etc.
[01:10:39] So you can see this is where sort of finance and economic, you know, socioeconomic background and wealth predicts, you know, educational outcomes. Because our education system was as dependent on, you know, educated parents or parents who can afford tutors, because a lot of the learning was done at home.
[01:10:56] So. Flipped Classroom comes along and says, hey, watch the videos, do this classroom stuff, you know, like, immersive stuff in the classroom. Love the idea. Problem was, teachers aren't really necessarily great at making videos. it's hard to track accountability on the videos, so kids wouldn't actually watch them.
[01:11:13] So there's a lot of, we just didn't quite crack that sort of user experience. Not for, you know, for student education, but I do think the concept there was good because I think it leads us into an AI future, right? Because I can imagine it's like, well, movement was really rethinking how information gets translated to students, how often students Get to interact and iterate.
[01:11:38] Oh, I failed, but a teacher was right there to help me. And then I did it, I did the next version again. I didn't have to do homework, turn it in, wait four days to get it back, and be like, Oh, oops, I totally misunderstood this. So my sort of cycle of learning is not five days, it's, you know, minutes. And I think that is the power that AI can, you know, can start to inject into our education system is, hey, as a student, I want to try this thing, and if I get it wrong, AI can help me right then and there.
[01:12:04] What we have not cracked is the, again, the user experience is like, well, students, everybody's a little bit lazy, so. Why try, like, do you, are we going to trust a student to watch the video with Flip Classroom, or are we going to trust the student now in AI sense? you know, don't ask the, don't ask the AI for the answer, ask it for help.
[01:12:25] and right now, I suspect we're seeing a lot of students like, oh, I'm just asking the AI for answers, like, hey, here, I'm just going to copy the whole, the whole question in, and it'll just tell me what to do. so there's a, an element of how we balance this is like, show your work, and be reflective, and We are now in a sort of an arms race with AI to how we validate, a student's learning and education, when homework, the struggle is the point, so if we, if we don't figure out how to encourage students to struggle at something and not just take the quick way out, which at this point is AI, we're never really gonna, I think we're gonna see a sort of a drop, a real drop off in educational outcomes, but it can, I think it can be solved, we just, We got to really reframe the whole home life, school life, the whole ecosystem has to, has to change pretty radically if we're going to make, acknowledge the existence of the AI in the system now.
[01:13:16] Marc Beckman: So, so, there are a few entrepreneurs that I know that are using, immersive experiences in the metaverse, VR specifically. There's a company, for example, I don't know if you're familiar with OptomaEd, but what they've done is they've recreated over 200 different experiences where students can now K 12, join, together with their professor.
[01:13:39] on the moon, at the Boston Tea Party, at the Great Pyramids of Egypt, and go through a, you know, several academic challenges. They need to, like, complete certain tasks with the professor. And what, Stanford has found is that these immersive experiences Increase the ability for the student to remember the lesson by as high as 75 to 80 percent.
[01:14:05] Whereas reading or lecturing to a student, the memorability of that experience is down at about 5 to 10 percent. So I think, this blending of perhaps spatial computing and virtual reality coupled with, immersive educational formats with tasks built in so the student has to go through to show that they've completed, that, that history class or the math or science or whatever it might be can, you know, be pretty much of a game changer.
[01:14:34] pretty interesting. So what we do, we've had you for a long time, James, today, and I really appreciate it, but a tradition that I have with every single guest is I like to finish the show, by, by setting up a, the beginning of a sentence and, and my guest completes it, it ties back into the name of the show, Some Future Day.
[01:14:53] So what I'd like to do is challenge you, I know this is just You know, off the cuff, but I'd like to challenge you, with the beginning of this sentence, since we're talking about children and education, in, in some future day, artificial intelligence will provide my children with a world where,
[01:15:11] James: a world where they can learn anything in a fun and engaging way and are then driven by their curiosity and excitement, which to me is the, the best way to learn anyway. So I think, I think AI can get us there. I, I, that's immersive, Experiences you were talking about. I think that that is the vision I, I hope for,
[01:15:39] Marc Beckman: Awesome. James, is there anything else that you'd like to add, before we sign off here today?
[01:15:46] James: I don't think so. This, I mean, thank you so much for, for having me on, on, this episode and this, this podcast. I, really enjoyed this conversation. We, we, we went a lot of different places. but it's always, it's always fun to tackle this. And I think, for all those out in the audience who interested in the AI space and wanna learn more, it is.
[01:16:06] I think one of the things that I would recognize is that AI is, AI is not a technology, at this point, and most people are not experienced at like, they're not going to be coding AI, they're going to be seeing it get its fingers in every little bit of their life, so just keep an eye out, just look, you know, look around you, start to acknowledge it, see what it can and cannot do, and just be, let's say, open minded.
[01:16:28] Be cognizant and versed in using AI as a as a consumer, and if If you do that, I think you'll be more prepared for the big changes that are coming, because you'll understand where it works really well and where it doesn't, because you've tried it. don't be afraid to just try it, don't be afraid to log in to some of these tools and give it a go.
[01:16:47] I think you'll, everyone will be surprised, delighted, confused, but everyone will learn, and that is, I think, the point.
[01:16:54] Marc Beckman: So dip your toe into the AI pool, James. Thank you so much. It's really been amazing speaking with you today.
[01:17:01] James: Yeah. Thank you so much.
[01:17:03]
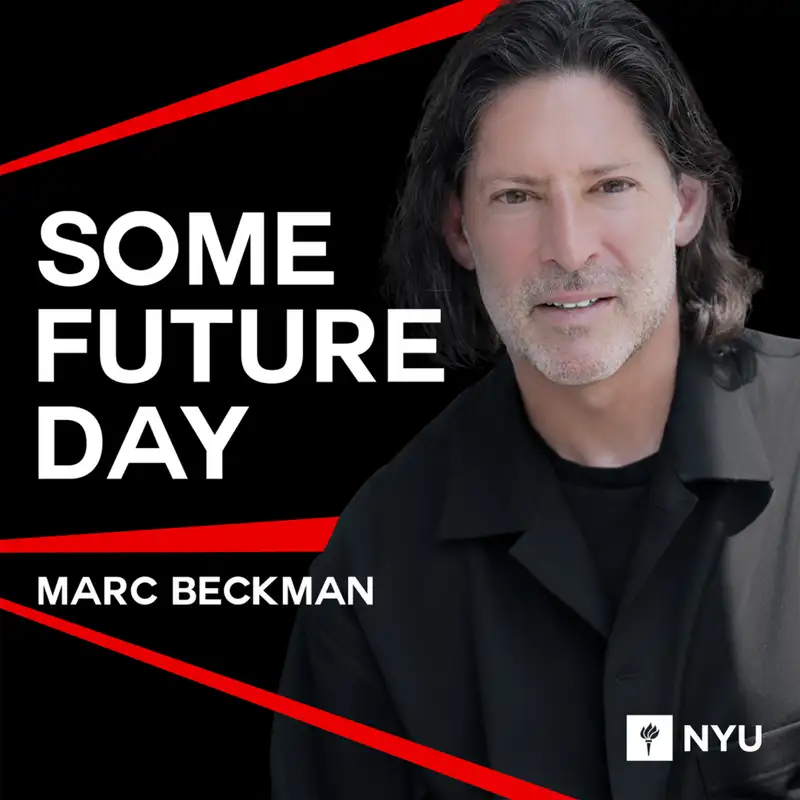